Analytics
The Whodunit of Big Data
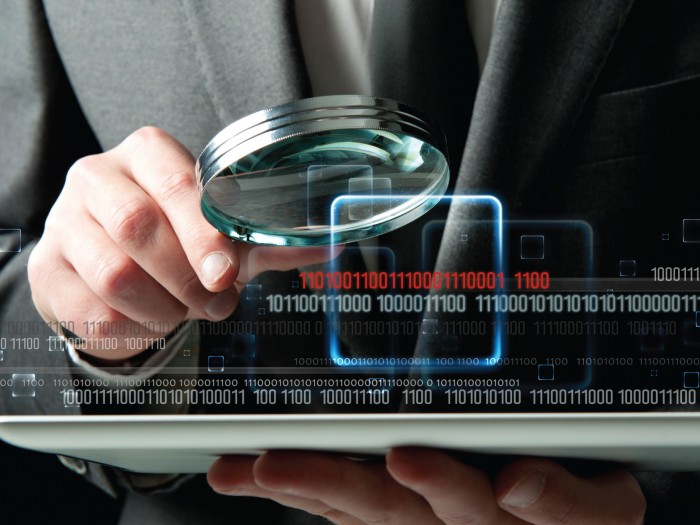
What do underwriters, actuaries, and claims adjusters have in common with police detectives, special investigators, and criminal profilers? One word: metadata. When a criminologist looks at geographic patterns or other characteristics associated with a crime, he or she first must know what data is available and, just as important, how that data is defined. The same is true for the actuary who attempts to identify risk-specific characteristics that may help more accurately underwrite and price a risk.
Metadata has gotten a lot of press lately — both good and bad. Authorities tracked down computer security pioneer and fugitive John McAfee through the metadata associated with a photo of him posted on a Central American online magazine blog. The digital photo included metadata — date, time, and geo-location — that pinpointed McAfee’s whereabouts.
The New York Police Department’s Collision Investigation Squad recently increased its efforts to understand and evaluate automobile crashes in New York City by using surveillance videos, cell phone records, vehicle black boxes, and mapping tools. The key to understanding those technologies and assessing their interoperability is metadata.
Contrast those metadata stories with the recent discussions about DNA, where the use of data and metadata may not be so clear-cut. State and local law enforcement agencies are amassing DNA databases. The Supreme Court recently ruled state authorities can collect DNA samples from people arrested for serious crimes. But some interpret that ruling as allowing local authorities to expand DNA collection to individuals beyond those associated with serious crimes and beyond jurisdictional borders.
Similarly controversial, the National Security Agency’s (NSA) efforts to compile metadata from phone and Internet records have resulted in a great debate. At the center of that issue is the interpretation of Section 215 of the PATRIOT Act, which permits the FBI to request “tangible things” to assist with an investigation. Those “things” include “books, records, papers, documents, and other items.” Some people, including the U.S. House of Representatives leadership, have labeled this information metadata.
Big data and, more important, the analytics that big data fuels are important emerging technologies. But as these real-life examples prove, metadata is really the key to unlocking analytics.
The next generation of devices and technologies will expand the amount of data available in the world, which then will expand the amount of data aggregated and analyzed. Social media and other new data sources, such as satellite imagery, video, voice, telematics, radio frequency identifiers (RFIDs), event data recorders (EDRs), and electronic health records (EHRs), are becoming more prevalent and accessible.
Much has been said about vehicle telematics and how that technology has changed the way insurers underwrite and price risk and administer claims. But think about human telematics and what that can do for life and health insurance, workers’ compensation, or loss control. Some hospitals are equipping patient wristbands with devices that can transmit blood pressure and heartbeat readings. And that’s just the start. Imagine devices as innocuous as a henna tattoo that can transmit the wearer’s bio-readings (blood pressure, heartbeat, enzyme and hormone levels, temperature, and so on) to machines that can interpret the information and identify warning signs of a heart attack or overexertion.
Imagine an RFID device “woven” into any product that can help trace the item’s movement through the supply chain from creation to modification to end product. How will that affect product liability and transportation risk?
Metadata: The Crucial Detail
But several questions remain: Will we have the metadata to support such discovery and analysis? What metadata do we need? And what do we mean by metadata?
The media have highlighted two different definitions of metadata: structural and descriptive.
The traditional data management definition of metadata relates to data structures and definitions. But metadata can also relate to descriptive information about, but not including, data content.
A standard envelope can illustrate the difference:
The traditional “structural” data management approach would define each data element and its attributes. A name and address may be a simple example, but in reality, they contain much more complex metadata, as follows:
* Sender name:
* Given name — 20 digits, alphabetic
* Surname — 20 digits, alphabetic
* State: U.S. — two digit alphabetic code defined by the U.S. Postal Service
* Postal Code: U.S. — five-digit numeric as defined by the U.S. Postal Service
So, structural metadata helps in understanding the format and definition of the information contained on the envelope.
From a “descriptive” metadata perspective, metadata would associate this “transaction” (the letter) with the actual envelope descriptors — John Doe to Jane Deer, addresses, when postmarked, by which post office, and so on — but like structural metadata, would not record the contents of the envelope.
So, what metadata do we need — structural, descriptive, or both? At the most basic level, we need structural metadata. A good detective must know the facts, develop them into clues, and make logical deductions from them. The task today is not only to gather data but, more important, to harness it in a way that produces value for the enterprise. And structural metadata is necessary to understand and interpret the facts.
Back to our examples: Without structural metadata, someone totally unfamiliar with the U.S. postal system would not know whether Jane Deer is the sender or recipient, whether Jane was the given or surname, or what the abbreviation SD means.
But descriptive metadata provides analytical value — a letter sent from John Doe in New York to Jane Deer in South Dakota.
Structural metadata is key to understanding data and defining and assessing its quality and purpose. It keeps the detective’s sources rich, cataloged, organized, and accessible.
Structural metadata facilitates getting the most value from data by providing the context for the data, thereby allowing data managers, data scientists, and all data users to understand and make proper use of data. And a well-constructed, accessible metadata repository supports knowledge sharing, facilitates research, and improves communication about data.
While structural metadata standards do exist for select applications (archiving, librarianship, records management), data sets (arts, biology, geography), and functions (education, government, science), there are no industrywide metadata standards specific to insurance. That does not mean the insurance industry is without metadata standards entirely — quite the opposite is true. Many industry organizations — ACORD, IAIABC, ANSI, WCIO, to name a few — have developed data element attributes that can form a basic metadata standard. However, no singular view exists across those organizations and within the industry.
Building upon the various data element standards used in the U.S. insurance industry, a base Insurance Metadata Element Set could include the elements listed in the table below.
Clues to Use
The insurance industry is using big data to analyze and solve problems associated with managing risk — risk-specific pricing, climate change, premium leakage, fraud, loss control, enterprise risk management, and so forth.
The job of the data manager is to make sure the main item needed to support those activities — data — is available, accessible, and of high quality.
But the most critical element of the data manager’s toolkit is structural metadata — the unifying element underlying all other toolkit functions. Without structural metadata, both descriptive metadata and, ultimately, the data content of the transaction, have no context.
Data management and analytics have much in common with detective work — both are a blend of art, science, and technology. Data managers collect, inventory, and organize the data.
Data scientists, actuaries, and statisticians are the detectives who develop the theories. Technologists provide the tools to process the data, and management provides direction and overall guidance.
Would that make Sherlock Holmes the ultimate data scientist?