AI Adoption Grows for Extreme Weather Risk Assessment
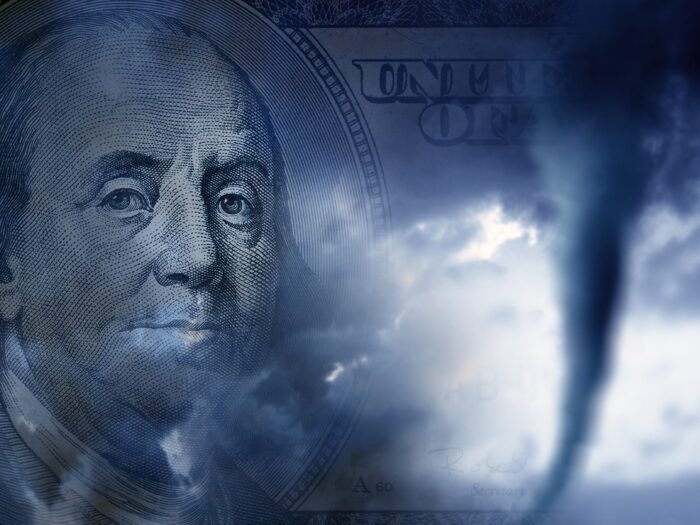
As natural catastrophes become more frequent and severe, a growing number of insurance companies are turning to artificial intelligence solutions for predicting and managing extreme weather risks.
A recent survey conducted by ZestyAI, involving over 200 property & casualty (P&C) insurance executives, explores current practices, the potential of AI-based models, and concerns surrounding transparency in the evolving landscape of climate risk management.
“AI has an incredible capacity to transform the insurance industry by enhancing the capability of carriers to protect the assets and wellbeing of policyholders in an increasingly complex world. This enthusiasm is reflected in our research — the consensus among insurance leaders is that AI will be a crucial enabler for realizing profitable growth going forward,” stated Attila Toth, founder and CEO of ZestyAI.
As insurers seek more accurate and proactive ways to predict and manage climate risk, three primary types of risk assessment models have emerged at the forefront:
- Traditional actuarial models, which have long been the industry standard, use mathematical and statistical techniques to project future risk based on historical claims data.
- Stochastic models take a different approach, simulating various outcomes under different conditions to provide a range of potential scenarios.
- The newest entrants to the field are AI and machine learning models, which are trained on unstructured data sources such as imagery, building permits, climatology data, and historical loss information to make predictions.
Usage Patterns Vary by Peril
Adoption of these models varies depending on the specific peril being assessed, ZestyAI reported. For wildfire risk, traditional actuarial models remain the most common tool, used by 54% of insurers. Stochastic models follow at 30%, while AI and machine learning-based models are used by 18% of companies for wildfire risk assessment.
The landscape shifts when it comes to severe convective storms. Here, stochastic models take the lead, employed by 45% of insurers. Traditional actuarial models are close behind at 42%, while AI and machine learning-based models are used by 23% of companies for this peril.
When it comes to the perceived accuracy of these models, there’s a notable lack of agreement on which type of model is most effective for predicting risk, the ZestyAI study found.
Traditional actuarial models are considered most accurate by 27% of industry professionals, while 26% favor stochastic models. AI/ML models are viewed as most accurate by 20% of respondents.
Interestingly, a significant portion of the industry – 27% – believes that a combination of different models offers the best risk prediction. This hybrid approach suggests a growing recognition that each model type has its strengths, and a multi-faceted approach may provide the most comprehensive risk assessment.
AI Adoption Trends Across Insurance Sectors
The embrace of AI technology is far from uniform across the insurance landscape, according to ZestyAI. Reinsurers and insurtechs are leading the charge, with 100% of respondents from these type of companies in agreement on AI’s benefits in managing climate-related losses. In contrast, national and regional carriers, along with farm bureaus, are more hesitant. Only 75% of national and regional carriers and 67% of farm bureaus recognize AI’s potential in this area.
When it comes to selecting AI or ML-powered models for risk assessment, insurers have clear priorities. The most important criteria include:
- Widespread adoption within the insurance industry (45%).
- Adoption by trusted peers (40%).
- Price of the model (37%)
Interestingly, factors such as regulatory approval (31%), proven ROI (27%), and model transparency (20%) rank lower on the list of priorities.
Perceived Advantages of AI Adoption
Despite varying adoption rates, there’s a growing consensus on the benefits of AI in insurance, the survey shows. A significant majority of insurance executives (80%) agree that AI and machine learning are opening new avenues for profitable growth. Moreover, 73% believe that AI models help better manage climate-related losses, and the same percentage agree that carriers adopting AI models will gain a competitive edge.
The use of AI models appears to correlate with higher confidence in managing climate risk. Among respondents using AI/ML models for severe convective storm risk, 81% felt they were ahead of the industry in adapting to climate-related challenges. This confidence level drops to 78% for those using stochastic models and 66% for those relying on traditional actuarial models.
Interestingly, confidence levels also vary by position within the company. Senior executives report higher confidence, with 75% of directors, 74% of vice presidents, and 73% of C-level officers believing their company is ahead of the industry in climate risk adaptation. In contrast, only 60% of managers and 64% of individual contributors share this level of confidence.
As the insurance industry grapples with evolving climate risks, transparency in risk assessment models has emerged as a critical concern. An overwhelming 90% of insurance executives agree that predictive risk models should be transparent.
This push for transparency extends beyond internal operations, with 79% of executives advocating for regulatory mandates requiring model transparency.
“The breakneck speed of Al advancements has the world and our industry running to keep pace. While regulations continue to grow and develop regarding Al usage in insurance, there are several things we already know to be true. Among them is transparency. Regulators are increasingly advocating for enhanced transparency and accuracy in how insurers assess risk,” the survey noted.
The benefits of transparency are twofold, serving both insurers and policyholders. For insurance companies, transparent models enhance their ability to communicate effectively with policyholders about potential risk mitigation strategies. This open dialogue fosters trust and collaboration between insurers and their clients.
On the policyholder side, transparency empowers individuals to take proactive steps in managing their property risks. By understanding the factors contributing to their risk assessment, policyholders can prioritize mitigation actions effectively, potentially reducing their overall risk profile and minimizing potential losses.
View the full survey here. &