Sponsored: Pinnacle Actuarial Resources Inc.
States Are Starting to Scrutinize Bias in Insurance Models. How Actuaries Can Help Carriers Evaluate AI and Data-Collection Fairness
Over the past few years, insurers have embraced the use of data collection, artificial intelligence (AI) and machine learning technologies in their underwriting practices. These tools allow insurers to more accurately identify loss trends and price risk, helping the industry to maintain profitability.
Though these tools have proved to be a valuable resource, insurers should scrutinize whether there are any unintentional biases lurking in their algorithms.
Researchers who have studied algorithmic bias have found that machine learning models can discriminate against people based on their race or gender, even if their creators did not intend for them to analyze those variables. If a tool scrapes social media or collects biometric data, for instance, it might inadvertently discriminate.
“The more information we use, the more we can indirectly represent a protected group,” said Gary Wang, senior consulting actuary, Pinnacle Actuarial Resources. “There’s a lot of information out there. An insurer can tap biometrics or things like social media-related information. With enough, we pretty much can know who the insureds are, and that includes their protected status.”
Colorado took the lead in ensuring these tools are unbiased, passing new regulations in 2021 that would require insurers to scrutinize what data they’re collecting to prevent discrimination against any protected classes, according to the National Law Review. The law requires that insurers evaluate their algorithms and update them to make sure they are using their data responsibly.
Colorado’s Law
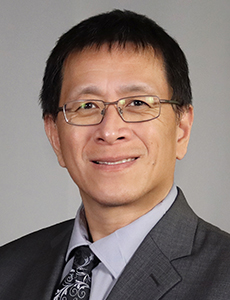
Gary Wang, Senior Consulting Actuary, Pinnacle Actuarial Resources
When underwriting policies, insurers look at a number of different data sources. Driving habits, geographic location and other factors can affect an insured’s exposure to various risks, so it’s important to consider these factors when pricing a policy.
Colorado’s law, Senate Bill (SB) 21-169, breaks the types of data insurers collect and feed into their algorithms into two categories: traditional and nontraditional.
Traditional factors include things like a policyholder’s loss history, the vehicle make, model and characteristics, and information about the driver such as driver age — the kind of data that has always been collected in the underwriting process.
Nontraditional data includes things like credit scores, social media habits, court records or level of educational attainment. The state is concerned that by collecting these types of data and using them in pricing algorithms, insurers could unintentionally discriminate against protected classes. It is important to note the distinction between the two categories of rating factors are not clearly defined and is still a work in progress.
Right now, Colorado’s law is still fairly broad. Insurers will need to implement company policies for how they use algorithms, and they will likely need to submit reports to regulators to ensure compliance with the new law. What exactly those processes will entail has yet to be determined.
“The regulation is still pretty broad and vague in scope, but you can definitely see that the policymakers want governance of some sort,” Wang said. “They want the companies to say we’ve done our due diligence.”
If Colorado’s law proves effective, other states may follow suit, implementing rules of their own to require them to assess their algorithms for bias.
Colorado’s regulation may even be used as a model for states and cities struggling to create detailed legislation around artificial intelligence. In January, New York City postponed implementation of a similar law that would regulate AI bias in a number of industries because it was too vague to be implemented, City and State of New York reported.
“I think it’s safe to say that if Colorado succeeds in any practical way, this will then become a blueprint for a lot of states to follow. I think other states are going to want to make sure this gets addressed in some way,” Wang said.
The ultimate goal of these laws is not to prevent insurers from using AI and machine learning algorithms in their pricing decisions but to ensure that these tools are being used fairly and don’t discriminate against any protected classes.
“All this is really to make fairness one of the priorities when we build models, when we price, when we deal with our policyholders, so that we are more proactively mindful that we are fair in how we treat them,” Wang said.
What Do These Regulations Mean for Insurers?
Given Colorado law, insurers will need to assess what kinds of data their models are analyzing and whether the algorithms they use have any unintentional biases. “When a company decides to use variables, when they decide to use models, they have to take the time to check if the models are fair,” Wang said.
Most insurers already avoid collecting a number of key variables, like race or income level, that could lead to accusations of discrimination against a protected class. Maintaining those best practices will be key as insurers evaluate what to do moving forward.
“We’re very careful to avoid variables and information about race, for example, or about income,” Wang said. “Our goal is simply to make sure we stay away from that information when we are trying to determine if somebody is a risky driver. Is somebody a good, safe household policyholder?”
Insurers should also work to analyze their models to see if any bias exists unintentionally. They can run tests or work with independent evaluators to determine whether their modeling system is in compliance with the new law. In addition to confirming compliance, these reviews can help carriers spot flaws in their algorithms and ultimately improve the pricing tools.
“We’re all looking at how we build a model. How does the model perform not just for overall accuracy, but for different groups of people?” Wang said. “Oftentimes, what this will mean is that we will have done a battery of tests and that, at least within these tests, we can show that we’ve gone through the checks and it looks reasonable.”
If carriers have their own research labs, they may opt to analyze their algorithms themselves: “Oftentimes, for the larger companies, they usually have a research lab, for example, so it’s just a matter of making it a priority,” Wang said.
How Actuaries Can Work to Help Ensure Fairness
Creating an open dialogue between carriers, lawmakers and consumer advocates will be key to helping insurers adjust to new regulations around AI and machine learning models.
“There’s a lot more discussion taking place now than there’s ever been,” Wang said. “The insurance companies, the regulators, the consumer advocates are all coming to the table talking about the concerns of where they think the lack of fairness is, where things might potentially be unfair.”
Pinnacle Actuarial Resources understands that the issue of discrimination in AI and machine learning underwriting models has a complex history. A leader in the predictive analytics space, the company has worked to develop ways to see whether models are accurate and treat insureds fairly.
“If we run models, what are we going to evaluate as the output? What will we look at? How will we summarize the information? What are the metrics we look at? We want to make sure what we’re looking at is relevant to the question that we’re asking,” Wang said. “We can help companies make sure that rates are accurate and fair.
Pinnacle Actuarial Resources can develop and analyze predictive models that satisfy multiple objectives. Pinnacle’s predictive analytics group can verify that a model predicts accurately and doesn’t include any unintentional biases.
“We have many different layers of checks that occur to make sure that our models meet the scope of the project,” Wang said. “As our projects reach their conclusion, we have the peer reviewer evaluate each model carefully and thoroughly to ensure key objectives are met.”
Pinnacle Actuarial Resources’ expertise in the predictive analytics space has positioned the company as a leader when it comes to understanding what data to collect and how to evaluate it. The company can serve as a critical resource as the industry adjusts to these new regulations.
“We understand what to look for and what to evaluate when we’re running these models,” Wang said. “We have become much more careful of evaluating the model — not just on the overall accuracy, overall correctness, but to make sure that there’s not hidden bias inside our models.”
To learn more, visit: https://www.pinnacleactuaries.com/.
This article was produced by the R&I Brand Studio, a unit of the advertising department of Risk & Insurance, in collaboration with Pinnacle Actuarial Resources, Inc. The editorial staff of Risk & Insurance had no role in its preparation.