6 Questions for Munich Re’s Michael Berger
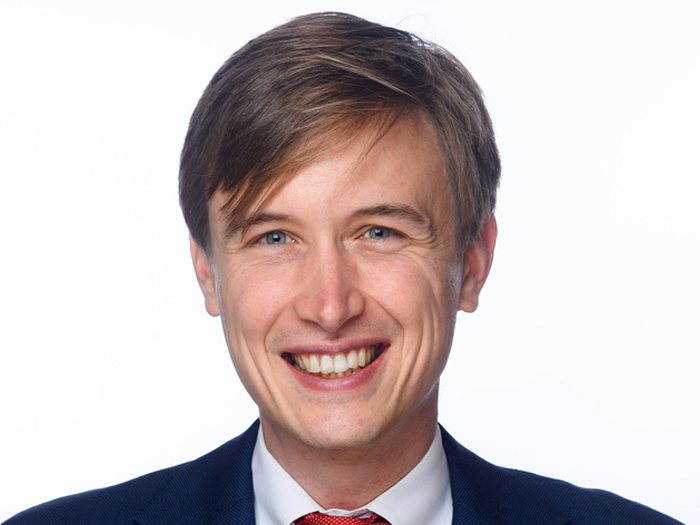
In February, Dan Reynolds, the editor-in-chief of Risk & Insurance, caught up with Michael Berger, the Palo Alto-based head of Insure AI at Munich Re. The topic of the conversation was the risks presented by generative AI and how underwriters are approaching that risk. What follows is a transcript of that discussion, edited for length and clarity.
Risk & Insurance: When we think about generative AI, there is a lot going on. But how do you see the risks that the technology presents?
Michael Berger: There are different risks that come with generative AI. Some of the risks are basically the same as with, let’s call it, normal artificial intelligence solutions and applications. So, for example, the risk of hallucination of a generative AI model. It’s the risk that the generative AI produces an output, for example a text, which might contain incorrect or misleading statements. This would be in a case where the user, with their prompt as input for the generative AI model, asked for a factually correct response.
This hallucination of the generative AI is just an error, and this kind of an error risk, we also see with any other AI application. Indeed, that’s a risk that we have already been insuring on my team since 2018. So, on this side, there’s nothing new conceptually — although of course with generative AI, one needs to look a little bit into the details on this hallucination risk.
Besides hallucination risk, there are also two big questions around copyright infringement: first when it comes to utilizing copyrighted assets, for example copyrighted images or copyrighted text in the training data of a generative AI model, and second when it comes to the output that the generative AI model produces.
This output might also be copyright infringing in the sense that the output is too close to a copyrighted asset which was used in the training data. A famous example here is the New York Times lawsuit against OpenAI and Microsoft, where the New York Times shows, in the generated text by ChatGPT, that there’s essentially a nearly one-to-one copy of certain New York Times articles.
There is another risk, which is basically the whole risk area of discrimination. So, discrimination in the context of generative AI might mean that discriminatory images are displayed, in the sense that certain groups are underrepresented or overrepresented with negative associations. There was, for example, an experiment done by the Washington Post where they looked into one generative AI model and they asked the model to produce portraits of people in certain situations.
When it came to depicting people at social services, those were predominantly displayed as either Asian or Black, while a “productive person” was displayed as a white male. So, we can clearly see this kind of discriminatory streak. When it comes to text, there might also be essentially discriminatory or inflammatory text that might be produced.
So, those are some of the “classic AI risks,” I would call them. There might be other risk considerations that we might need to take into account when it comes to generative AI models. One is clearly that the generative AI model is usually a very big model. It takes a lot of computational resources to train those kinds of models. As we know from discussions around data centers, there are environmental concerns to consider.
In this environmental context comes another risk: What impact, in terms of energy consumption or water consumption, do we see as a result of training those models and also in updating them? If a model will need to be updated more often than expected, for example because its usefulness or its accuracy degrades faster than expected, then of course we need to train it more, so we have increased computational and energy-related costs, but we also have a higher-than-expected impact on the environment.
R&I: And Munich Re, as you said, has been covering some artificial intelligence since 2018. What actions or circumstances have triggered claims in this area?
MB: One is simply randomness. There wasn’t anything done wrong by the data science team, nor was there any systematic change in the underlying data. But by chance, a model — and this was in the financial fraud detection space — had seen more difficult examples compared to the number of difficult examples that were in the training data. Basically, there was a performance drop of the model, and this resulted in a claim.
But that’s what Munich Re is in the business for. So this kind of natural randomness, the natural randomness of the performance fluctuation, that’s what we also intend to take on.
The interesting thing here is that basically every AI system, including every generative AI system, is just a probabilistic system. So this means, even if you build the most perfect AI or generative AI model, there will always be a probability that the AI or GenAI makes mistakes or hallucinates. This cannot be technically avoided. Always the residual risk remains, this residual randomness of the AI making errors or GenAI hallucinating. That’s basically the risk that we are taking on.
Another claim situation that Munich Re has seen is really a systematic change in the underlying data — basically, where there was this kind of change between the output and the respective input for the model, and then the AI system on the monitoring and retraining side did not pick up this change fast enough. Therefore a performance drop occurred, and it took our client a couple of months to restore the performance of the model again. During this time, the client incurred financial losses because the AI was used in automated decision-making, and the decisions were then wrong more often than expected, and this cost them financially.
We haven’t really seen an AI loss that was due to the data science team making a mistake. It was really always either the statistical randomness itself or the change in underlying data which triggered a loss.
R&I: When we think about possible exposures or occurrences or losses, what insurance lines come into play?
MB: It’s a tough question, because it can touch on almost every line. This is the reason our Munich Re AI insurance product is a stand-alone insurance product. For the error risk of the AI, we have to sell our own product, which is triggered if the AI either makes the first error — so each error, it’s triggered — or a certain kind of error-rate statistic falls below a certain threshold or exceeds a certain threshold, then it’s also triggered, regardless of what the underlying reason is.
We designed our solution like that because, for us, this is the most suitable cover concept for covering this inherent risk that comes with AI. We wanted to address the basic question, “Does the AI really consistently perform well?” To answer this question, we designed this stand-alone AI performance insurance product.
However, for other risks, there might be certain kinds of liability situations, risk situations, which might also be covered under traditional insurance product. Either silently, so that it just falls into it — whether it’s by design that a policy stays silent or it was an oversight — or for certain situations, it’s really the intention that it is admittedly covered there.
For example, if a cyberattack happens and the model is shut down by the cyberattack, business interruption losses can occur, and this is a classical cyber insurance business interruption claim. This risk falls into the intended scope and design of cyber insurance with business interruption.
However, there are other risk situations which could touch on traditional insurance solutions. In those instances, insurer and underwriter need to be careful whether the risk should really be covered there and whether the underwriting and pricing needs to change if we want to cover those specific risks with traditional insurance products to effectively account for the changing exposure, the changing risk that exists.
R&I: When you think about Munich Re’s approach, as you mentioned, the company’s been underwriting AI since 2018. Would you say this is a business that you’ve got some type of appetite for? How would you characterize that?
MB: In terms of your point about a gap versus an overlap, for the error risk, we basically see it as a gap. Because from our perspective, the error fluctuation risk of the AI, that’s just inherent to the AI model. It doesn’t require any kind of negligence for a loss to occur. This is the reason why we’ve designed this stand-alone cover to serve this kind of demand for financial protection from AI model developers and model users, and, from our perspective, to address the clear gap that is there.
From an appetite perspective, we see performance covers for AI as a very interesting growth area, because more and more companies are experimenting with AI across industries, across sizes, from traditional companies to tech companies. Their intention, of course, with AI is that they are using it either as a system to support decision-making or to automate certain decisions and certain processes.
This means that companies are relying on the output and the accuracy of the AI models. So basically, for all of them using those AI models, there’s this inherent error uncertainty present. Munich Re sees this AI insurance as a strong area of growth with a lot of potential. My team is able to insure this kind of AI error risk across domains, across industries, as insurers or as reinsurers, taking up this kind of a risk. This is, for Munich Re, a very, very interesting area.
Besides the error risk, there are also other risks out there, as I mentioned at the beginning. For generative AI, we look at copyright infringement risks and the risk of discrimination. For both of those scenarios, we are working with current clients in structuring specialized insurance solutions.
To underwrite and price this kind of risk, my team can find an interesting translation, for example, translating the discrimination risk into some form of an error risk of the AI — so, the error that an AI makes in terms of some kind of fairness or discrimination metric. It basically becomes an error risk again. Then we can use the underwriting, the pricing platforms, what we have developed at Munich Re for the insurance of the error, to then expand into the insurance of discrimination risk. This is how we think about it, from the error insurance, to build on our initial Munich Re product to also encompass other classical AI and generative AI risks.
R&I: When you look at the insurance landscape — because Munich Re is, of course, a reinsurer — what do you see in terms of capacity generally for this sector? Could you quantify that for us or describe it?
MB: I can talk about the market in general. We’re the leading and one of very few insurers out there really offering a dedicated AI insurance product. There are also other players out there. We know from our conversations with other insurance companies that they are also are looking into the space, and they’re also looking into our products there as well. So we see that there’s also an interest to participate in this attractive growth field from a wider community perspective.
Currently, we are really acting through our own primary insurance companies within Munich Re Group, but we’re also open to working on the reinsurance side. So this is also a viable business possibility for us. Structurally, we basically are able to play on both sides and essentially bring the expertise that we have developed in doing this AI insurance business for more than five years now also to the reinsurance side.
R&I: Is there anything about this topic, either on the risk side or the coverage side or the market side, that we didn’t discuss or that I didn’t ask you about?
MB: From my team’s side, we are working in two geographic locations. Half of my team is here in the U.S. The other half of the team is based in Munich.
We have set it up like that because where we see the most demand is in the U.S., Europe, with the London market, and also Israel. In Israel, especially the tech companies there and the companies in the financial fraud detection space, the cybersecurity space, they’re building some interesting AI models. We are seeing demand there.
Although, I must say that we have also seen demand from India as well as Japan, and we are pleased about this, even if those are not our key focus areas. So, it’s really the U.S., Europe and Israel as the main focus. &